Researchers at MIT's Improbable AI Lab and the Institute of AI and Fundamental Interactions have been working on ways to improve a robotic mini-cheetah's strides. Their model-free reinforcement learning system helped the legged robot break the record for the fastest run recorded.
The mini-cheetah has an indoor sprint of 3.9 meters per second (8.7 miles per hour), an outdoor 10-meter dash of 3.4 m/s (7.6 mph), and an indoor spin of 5.7 radians per second, according to the project's website.
MIT News spoke to Gabriel Margolis, a Ph.D. student at MIT Computer Science and Artificial Intelligence Laboratory (CSAIL), and Ge Yang, a postdoctoral fellow at the Institute of AI and Fundamental Interactions (IAIFI), about just how fast the quadruped robot can run.
Q: We’ve seen videos of robots running before—why is running harder than walking?
Achieving fast running requires pushing the hardware to its limits, for example by operating near the maximum torque output of motors. In such conditions, the robot dynamics are hard to analytically model.
The robot needs to respond quickly to changes in the environment, such as the moment it encounters ice while running on grass.
If the robot is walking, it is moving slowly, and the presence of snow is not typically an issue. Imagine if you were walking slowly, but carefully. You can traverse almost any terrain.
Today’s robots face an analogous problem. The problem is that moving on all terrains as if you were walking on ice is very inefficient but is common among today’s robots. Humans run fast on grass and slow down on ice—we adapt.
Giving robots a similar capability to adapt requires quick identification of terrain changes and quickly adapting to prevent the robot from falling over.
In summary, because it’s impractical to build analytical— human-designed—models of all possible terrains in advance, and the robot's dynamics become more complex at high-velocities, high-speed running is more challenging than walking.
Q: Previous agile running controllers for the MIT Cheetah 3 and mini-cheetah, as well as for Boston Dynamics’ robots, are “analytically designed,” relying on human engineers to analyze the physics of locomotion, formulate efficient abstractions, and implement a specialized hierarchy of controllers to make the robot balance and run. You use a “learn by experience model” for running instead of programming it. Why?
Programming how a robot should act in every possible situation is simply very hard. The process is tedious, because if a robot were to fail on a particular terrain, a human engineer would need to identify the cause of failure and manually adapt the robot controller, and this process can require substantial human time.
Learning by trial and error removes the need for a human to specify precisely how the robot should behave in every situation. This would work if: (i) the robot can experience an extremely wide range of terrains; and (ii) the robot can automatically improve its behavior with experience.
Thanks to modern simulation tools, our robot can accumulate 100 days’ worth of experience on diverse terrains in just three hours of actual time.
We developed an approach by which the robot’s behavior improves from simulated experience, and our approach critically also enables successful deployment of those learned behaviors in the real-world.
The intuition behind why the robot’s running skills work well in the real world is: Of all the environments it sees in this simulator, some will teach the robot skills that are useful in the real world.
When operating in the real world, our controller identifies and executes the relevant skills in real time.
Q: Can this approach be scaled beyond the mini-cheetah? What excites you about its future applications?
At the heart of artificial intelligence research is the tradeoff between what the human needs to build in (nature) and what the machine can learn on its own (nurture). The traditional paradigm in robotics is that humans tell the robot both what task to do and how to do it.
The problem is that such a framework is not scalable, because it would take immense human engineering effort to manually program a robot with the skills to operate in many diverse environments. A more practical way to build a robot with many diverse skills is to tell the robot what to do and let it figure out the how.
Our system is an example of this. In our lab, we’ve begun to apply this paradigm to other robotic systems, including hands that can pick up and manipulate many different objects.
Article topics
Email Sign Up
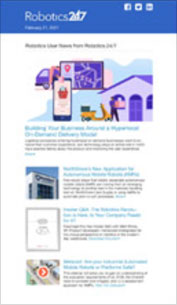
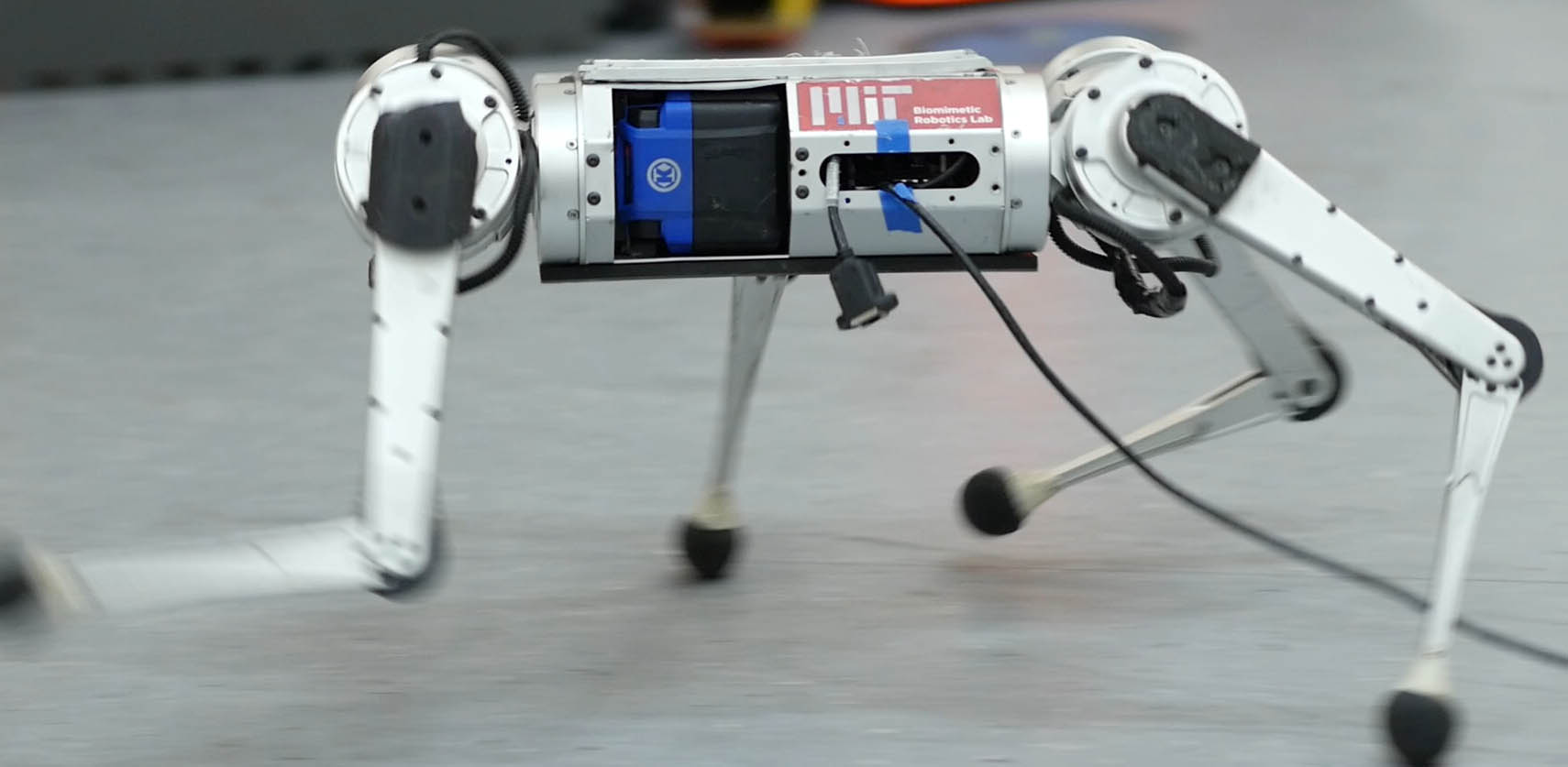