Carnegie Mellon University Engineering and Public Policy (EPP) Ph.D. student Prithvi Acharya and his advisor, Civil and Environmental Engineering’s Scott Matthews, teamed up with EPP’s Paul Fischbeck. They have created a new method for identifying over-emitting vehicles using remote data transmission and machine learning that would be less expensive and more effective than current inspection/maintenance (I/M) programs, according to the university.
In an attempt to eliminate unnecessary costs and improve the effectiveness of I/M programs, Acharya, Matthews and Fischbeck published their recent study in IEEE Transactions on Intelligent Transportation Systems.
Their new method entails sending data directly from the vehicle to a cloud server managed by the state or county within which the driver lives, eliminating the need for them to come in for regular inspections. Instead, the data would be run through machine learning algorithms that identify trends in the data and codes prevalent among over-emitting vehicles. This means that most drivers would never need to report to an inspection site unless their vehicle’s data indicates that it’s likely over-emitting, at which point they could be contacted to come in for further inspection and maintenance.
The team’s work has shown that much time and cost could be saved through smarter emissions inspecting programs, but their study has also shown how these methods are more effective. Their model for identifying vehicles likely to be over-emitting was reportedly more accurate than current on-board diagnostics systems, according to the university.
Sources: Press materials received from the company and additional information gleaned from the company’s website.
About the Author
Press releases may be sent to them via [email protected]. Follow Robotics 24/7 on Facebook
Follow Robotics 24/7 on Linkedin
Article topics
Email Sign Up
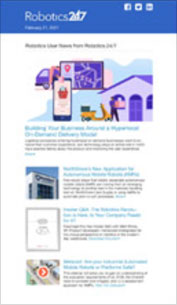