With all the interest lately in artificial intelligence, robotics, and ethics, it's worth noting some of the ongoing research in the field. The Allen Institute for Artificial Intelligence, or AI2, last week announced Ali Farhadi as its new CEO. He is an AI researcher, executive, and Forbes Top 5 AI entrepreneur, said the organization. It also presented the Phone2Proc robot training approach.
“As we face unprecedented changes in the development and usage of AI, I could not think of a better time to return to AI2 as CEO,” stated Farhadi in a blog post. “Today more than ever, the world needs truly open and transparent AI research that is grounded in science and a place where data, algorithms, and models are open and available to all.”
“I believe this radical approach to openness is essential for building the next generation of AI,” he said. “The world-class researchers and engineers at AI2 are uniquely positioned to lead this new open and trusted approach to AI development.”
Farhadi returns to AI2 from Apple
Founded in 2014 by late Microsoft co-founder Paul G. Allen, the Allen Institute for Artificial Intelligence is a nonprofit involved in foundational and generative AI research. The organization said it employs more than 200 researchers and engineers from around the world, “taking a results-oriented approach to drive fundamental advances in science, medicine, and conservation through AI.”
Farhadi is a professor at the Paul G. Allen School of Computer Science & Engineering at the University of Washington, where he has won several best paper awards.
In 2015, Farhadi started AI2's Computer Vision team, and he co-founded Xnor.ai, an on-device deep learning startup that Apple Inc. acquired in 2020. Farhadi rejoined the Seattle-based AI2 from Apple, where he led its next-generation machine learning efforts.
Allen Institute for AI presents Phone2Proc
At last week's Conference on Computer Vision and Pattern Recognition (CVPR), held by the Institute of Electrical and Electronics Engineers (IEEE) and the Computer Vision Foundation in Vancouver, Canada, AI2 presented a paper on Phone2Proc. The approach involves scanning a space, creating a simulated environment, and procedurally generating thousands of scenes to train a robot for possible scenarios.
AI2 claimed that Phone2Proc can more easily and quickly train robots to perform in settings such as a floor covered with toys, a room whose furniture has been moved, or a cluttered workspace.
Robotics 24/7 spoke with Kiana Ehsani, one of the researchers leading the Phone2Proc effort. She studied under Farhadi at the Paul G. Allen School of Computer Science and said she focuses on computer vision and embodied AI for training agents to interact with their environments.
How can AI make robots behave more intelligently?
Ehsani: We're still very far from robust robots. We don’t yet have agents that can work in any house or generalize behavior like large language models.
Even relatively simple robots like the Roomba work in just two dimensions. Generalization is different in other domains of AI than robotics. As a customer, I don't care how a robot can generalize; I care if it can be robust in my specific environment.
By creating training models in different environments and pretraining, we can generalize AI to other environments and fine-tune it. By sampling trajectories, we can build better training models.
In the case of a household robot, for example, by training it in a specific house, it gets better in a learning environment. It's like introducing houseguests, but doing in the physical world is unsafe, costly, and slow.
How will you make it easier to train robots?
Ehsani: What if I could walk around a room with my smartphone, push a button, and then calibrate? We can take a quick video, and Phone2Proc will generate an environmental template automatically.
It will procedurally generate themes specific to the house and environment in simulation. It will have the same layout but not exactly the same furniture and lighting. By generating thousands of scenes, training is safer, cheaper, and faster.
We compared our methods with the state of the art. We thought we could achieve these improvements, not just in one setting, but also training in a practical way for lighting changes, moving furniture around, or people moving around.
Training robots from simulation to reality
We've heard a lot about the metaverse lately. How can simulation account for random objects on its own?
Ehsani: By using that scan, we get the walls and 3D bounding boxes around the objects. In one example, Phone2Proc looks at a black and white image and then builds a template.
It can then randomly choose walls, flooring, and any object of a type that has been scanned—for example, a variety of sofas. It learns to detect unorthodox sofas, and we can randomize lighting and textures and randomly place different objects.
Because we have a procedural way of generating scenes, it can randomly select objects with no human in the loop.
How does Phone2Proc handle semantic understandings of objects?
Ehsani: The library has annotations. There's parallel work in the Objaverse.
We've expanded our asset library by a significant amount. We can sample annotations of object types and put them in an environment. We have a procedural way of the affordances, like the likelihood of finding an apple on a table is higher than finding one on a bed.
Does Phone2Proc account for people in simulations?
Ehsani: We don't yet have any simulated people or dynamic figures—that's a topic for future work. We are training robots by having different objects in their way, so they can find ways to get aound them.
There are multiple ways of reaching the same place, like corridors. In each run of an experienment, there's a new object in the location, which requires the robot to adapt to changes in its environment and update its path planning.
What types of robots is the Allen Institute for Artificial Intelligence training?
Ehsani: This work is only with open-source LoCoBots for navigation. We've looked at some use cases for finding a specific object in the house, such as keys.
In simulated environments, they’re all fully interactive. We're training mobile manipulation agents in one project, where they're moving objects and briniging or rearranging stuff.
In simulation, we've seen various robot models. Do you build 3D models of each, and how does that translate back to physical robots?
Ehsani: They're put into the Unity development platform. We have built a Python package on top of a ROS package to bridge the simulation-to-reality gap.
The APIs used for training in simulation or in the real world are similar. We make them as close as possible, and it's absolutely possible to train in both reality and simulation.
The physics realism is fully tractable. We have annotated the objects' masses, and Unity has a built-in physics engine to simulate interactions.
If agent’s arm hits an object, it wil have an effect on it. It’s very important for an agent to learn the consequences to its actions to be safe.
How many objects are in your library?
Ehsani: That's more challenging. We've been working on it, and we have 100 types of sofas, 10 to 15 types of vases, and samples for each category.
The Objaverse has 800,000 different types of objects. For scaling, our projects are aligned, and it can combine with Phone2Prog for visual diversity.
Research could lead to more helpful robots
You mentioned mobile manipulation. How can that be used?
Ehsani: We are working on using simulated environments to do object manipulation, which important for assisted living facilities.
We're also working on generating large-scale data sets that can be used by the entire community. We have simulated environments, and we have a lot of sensory inputs. Phone2Proc enables a lot of data collection that was not possible before.
What are your expectations for this research?
Ehsani: With our CVPR submission, we have shared some results. We're hoping that in the span of the next year, we'll have robotic manipulation agents, which is an achievable goal.
One of the most challenging areas of the home for robots is the kitchen, so that's one of the areas we're focusing on.
Just learning how a refrigerator door opens—vertically versus horizontally—this project can help the community.
We could adapt Phone2Proc to agents to more easily learn the specific characteristics of any environment, and then try out all variations.
About the Author

Follow Robotics 24/7 on Linkedin
Article topics
Email Sign Up
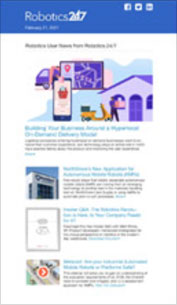
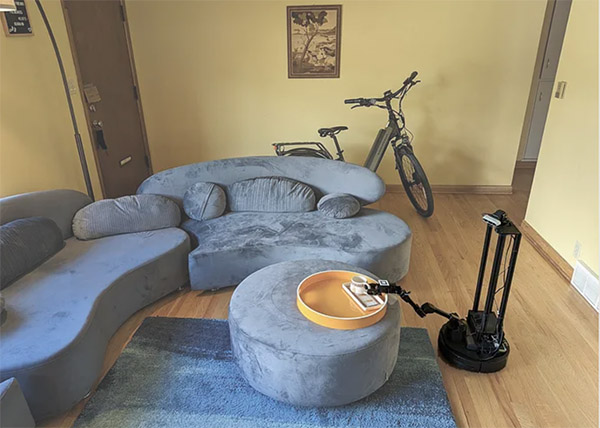