Covariant, a California-based AI Robotics company, released RFM-1 at MODEX 2024, a Robotics Foundation Model that provides robots the human-like ability to reason. The RFM-1 represents the first time generative AI has successfully given commercial robots a deeper understanding of language and the physical world, according to the company.
Covariant offered show attendees an experience of RFM-1. The company also encouraged interested parties to book a live demonstration at the company’s headquarters in Emeryville, Calif.
Challenges with robotic automation, AI
The key challenge with traditional robotic automation and automation based on manual programming or specialized learned models is the lack of reliability and flexibility in real-world scenarios. To create value at scale, robots must understand how to manipulate an unlimited array of items and scenarios autonomously.
By starting with warehouse pick and place operations, Covariant’s RFM-1 showcases the power of Robotics Foundation Models. In warehouse environments, the company’s approach of combining the largest real-world robot production dataset with a collection of internet data. The approach helps open new levels of robotic productivity and shows a path to broader industry applications, ranging from hospitals and homes to factories, stores, restaurants and more.
“Robotics Foundation Models require access to a vast amount of high-quality multimodal data,” said Peter Chen, CEO and co-founder at Covariant. “These models require data that reflects the wide range of information a robot needs to make decisions, including text, images, video, physical measurements and robot actions.”
Since 2017, Covariant’s previous AI models have enabled robots to operate in a commercially meaningful way across a diverse set of warehouse operations and industries. These robots have been able to adapt to their environment, understand the scenes they are faced with, reliably handle items they have never seen before and achieve human-level speed and reliability.
The introduction of RFM-1, according to the company, opens new doors for what’s possible with these robots. RFM-1 is set up as a multimodal Any-to-Any sequence model, and is an 8-billion parameter model that is trained on text, images, video, robot actions and physical measurements to autoregressively perform next-token prediction. Because it tokenizes all modalities into a common space, the next-token prediction training enables RFM-1 to understand any modalities as input and predict any modalities as output.
“Unlike AI for the digital world, there is no internet to scrape for large-scale robot interaction data with the physical world,” Chen added. “So, we built a highly scalable data collection system which has collected tens of millions of trajectories by deploying a large fleet of warehouse automation robots to dozens of customers around the world.”
With a deep understanding of language and the physical world, RFM-1 gives robots the sophisticated ability to reason and make decisions on the fly. This delivers, according to Covariant, high levels of robotic autonomy, lowers associated costs and implementation times, and opens the door for the rapid development of new applications and robotic form factors = such as consumer and humanoid robots.
RFM-1 capabilities
Specific RFM-1 capabilities include:
- Physics world model: RFM-1’s understanding of physics emerges from learning to generate videos. RFM-1 can predict via AI-generated videos how objects will react to robotic actions. This physics world model, powered by Covariant’s multimodal robotics dataset, improves speed and reliability by enabling robots to simulate the result of future scenarios and select the best course of action.
- Language-guided programming: By making robots taskable and giving them an understanding of the English language, RFM-1 enables robots and humans to collaborate and problem-solve by simply communicating with each other - lowering the barriers of customizing AI behavior to address dynamic business needs and the long-tail of corner case scenarios.
- Learning from self-reflection: In-context learning allows robots to learn on the fly and improve based on the self-reflection of their own actions. With RFM-1, robots can realize this learning in minutes - as opposed to weeks or months - which increases performance while reducing ramp time for a new system, scenario, or item.
“Recent advances in generative AI have demonstrated beautiful video creation capabilities, yet these models are still very disconnected from physical reality and limited in their ability to understand the world robots are faced with,” said Pieter Abbeel, chief scientist and co-founder at Covariant. “Covariant’s RFM-1, which is trained on a very large dataset that is rich in physical robot interactions, represents a significant leap forward towards building generalized AI models that can accurately simulate the physical world.”
Article topics
Email Sign Up
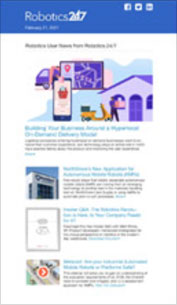
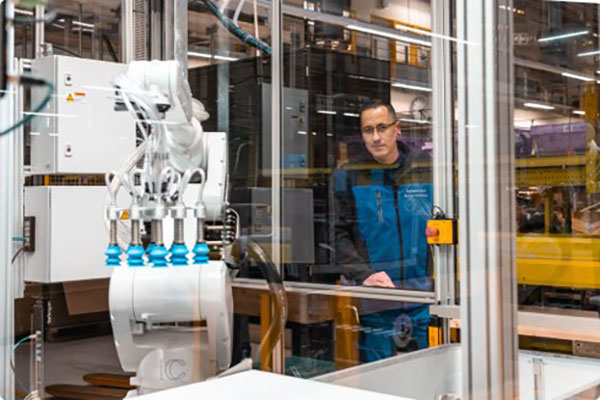